Breaking Down Data Silos for Business Success
Data, AI, and analytics “are fragmented ecosystems of priorities, goals, operations, talent, information, and technology,” writes Forrester Research in a recent report. Despite their theoretical interconnectedness, these capabilities often operate in silos, creating barriers to achieving business objectives.
Forrester Research’s October 2024 report “Unify Data, AI, And Analytics To Activate Business Outcomes”, highlights the prevalence of data silos as a significant challenge for global data and analytics decision-makers. To overcome this, a cohesive strategy that integrates capabilities and aligns enterprise, business unit, and individual interests is vital. Here’s our summary of the Forrester report on how to unify your data, AI, and analytics capabilities to drive business success.
Scaling Data and AI Across the Enterprise
To effectively scale data, AI, and analytics, start with clear objectives aimed at amplifying business outcomes.
Consider how to create scale regardless of the solution’s origin, whether it’s an enterprise initiative, a business unit solution, or grassroots individual use:
Clear Objectives: Establish clear, ambitious goals that align with business outcomes.
Unified Infrastructure: Coordinate both soft (organizational readiness) and hard (technical foundation) capabilities.
Increased Investment: “45% of global technology decision-makers working in a data discipline say their organizations are increasing budgets for data, analytics, governance, and AI by 5% or more in the next twelve months,” according to Forrester Research.
By aligning resources and investment strategies across the organization, you can ensure a scalable and effective data, AI, and analytics competency.
Your Data. Supercharged in 30 Days.
Start your free trial of Strategy One Standard—where AI meets BI to deliver fast, secure, enterprise-ready insights.
Enabling Data Literacy and Self-Service
A key to successful implementation is preparing the workforce for wide adoption. Forrester indicates that “literacy is insufficient and investments fall flat without centers of excellence and platforms to enable self-service.”
Focus on:
AI Literacy Training: Integrate AI literacy and training plans into your AI strategy.
Fostering Expertise: Develop the workforce’s data literacy to advanced levels.
Self-Service Platforms: Facilitate high adoption rates by providing a quality user experience and enabling self-service.
Ensure investments in data, AI, and analytics turn into substantial business value by prioritizing user enablement and literacy.
Read the full report
Forrester Research: Unify Data, AI, And Analytics to Activate Business Outcomes
Building Trust in Data Systems
To achieve reliable and actionable insights, organizations must build trust in their data systems. “Concerns over generative AI (genAI) hallucinations, cyber attacks, and regulations are front and center for enterprises,” notes Forrester Research.
Address these concerns by:
Improving Data Quality: Focus on enhancing data quality and reliability as part of data governance.
Introducing Responsible AI Training: Implement responsible AI standards and training for all AI programs.
Ensuring System Reliability: Develop systems and processes that align with enterprise values and ethics, ensuring they are observable and reliable.
This approach ensures that information, insights, and intelligence are trustworthy and actionable.
While the Forrester Research report offers a critical lens on the fragmentation of data, AI, and analytics—and the organizational strategies needed to overcome it—the practical path forward often requires specialized tools and frameworks tailored to an enterprise’s unique environment.
At Strategy, we’ve translated these insights into action through our own innovations. Lat’s take a look at how Strategy’s Semantic Graph operationalizes many of the principles outlined in the report, offering a scalable, privacy-aware, and unifying data architecture designed to meet the real-world needs of modern enterprises.
Leveraging Strategy’s Semantic Graph to Unify Data
Strategy’s Semantic Graph exemplifies an advanced data management tool designed for unifying data across an organization. Built on core principles of reusability, inheritability, and privacy awareness, it streamlines data processes and aligns them with organizational objectives.
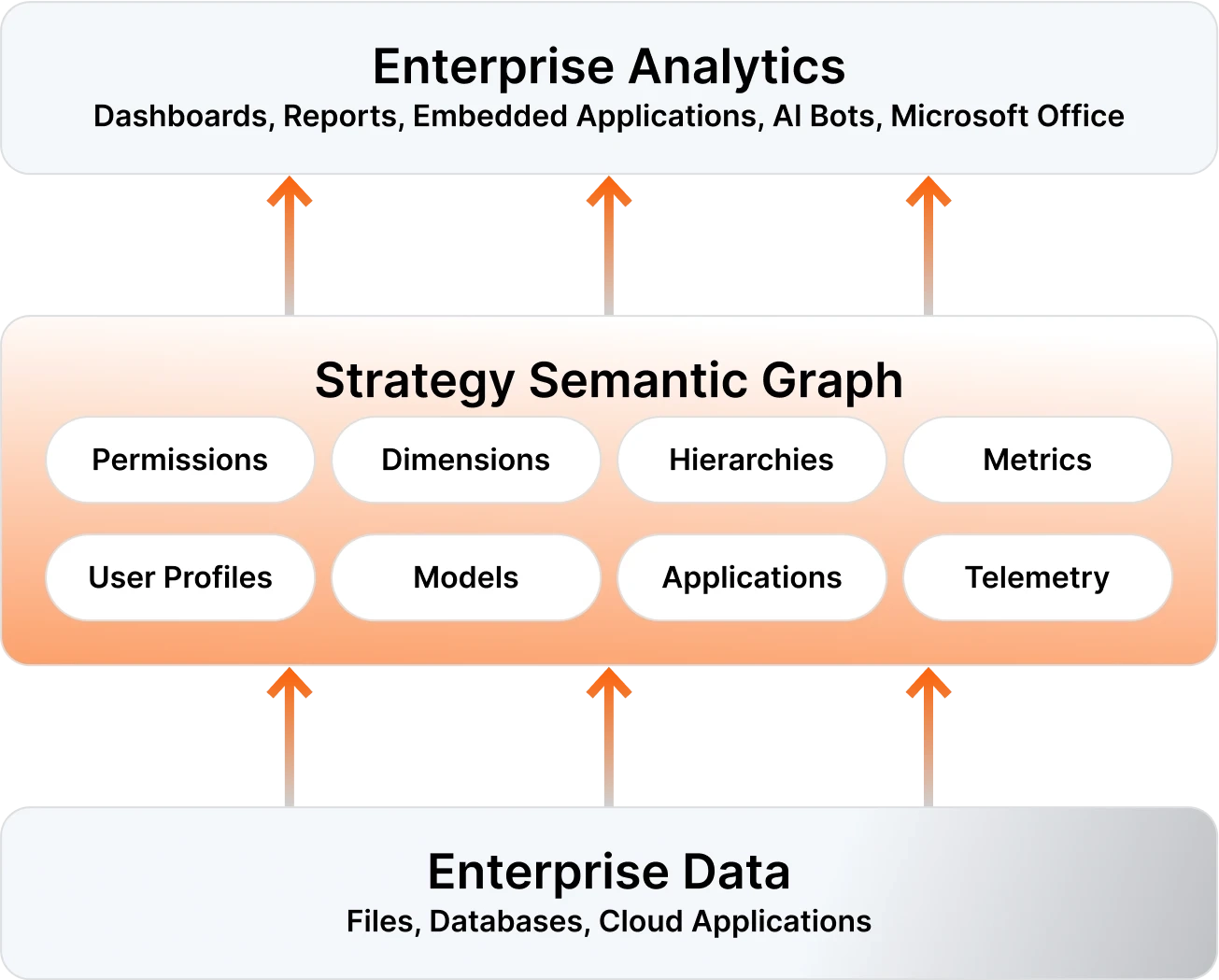
Reusable Data Objects
The Semantic Graph features reusable data objects to significantly reduce development time, allowing teams to focus on innovation. By delivering consistent reports and applications across various departments, the Semantic Graph ensures coherence in data interpretation, fostering an ecosystem where data use becomes standardized and reliable.
Inheritable Updates
When updates are made to a core object or rule, the Semantic Graph propagates these updates automatically to all dependent objects. This inheritability ensures that any changes are immediately reflected throughout the organization, maintaining consistency, reducing maintenance costs, and keeping the entire data ecosystem current and reliable.
Privacy-Aware Architecture
Data privacy is crucial, and the Semantic Graph enforces stringent role-based access controls and privacy rules. This ensures compliance with privacy regulations and safeguards sensitive information. As a result, users can rely on the integrity and security of the data available to them.
Platform Agnosticism and Scalability
The Semantic Graph’s platform-agnostic nature ensures flexibility and scalability, integrating seamlessly with various cloud providers and on-premise environments. This adaptability allows organizations to expand their data management practices without compromising performance or security.
Centralized Governance and Robust Security
By centralizing data governance, the Semantic Graph eliminates data silos, offering a single source of truth for all business data. Robust security measures, including encryption, authentication, and user permissions, ensure secure data access and protection from threats.
Cost Efficiency
The Semantic Graph enhances cost efficiency by reducing IT overhead through its reusable structures and self-service analytics capabilities. This reduction in maintenance costs translates to significant savings across the organization.
Unifying data across an organization is critical for driving business success. By scaling data, AI, and analytics capabilities, enabling broad adoption, and building trustworthy systems, organizations can overcome fragmentation. Leveraging tools like Strategy’s Semantic Graph can further unify data, ensuring reliability, security, and efficiency, thus fostering a data-driven culture poised for innovation and growth.
Read more about breaking down data silos in the Forrester Research report “Unify Data, AI, And Analytics To Activate Business Outcomes,” courtesy of Strategy.